搜索结果: 1-14 共查到“数理统计学 Learning”相关记录14条 . 查询时间(0.128 秒)
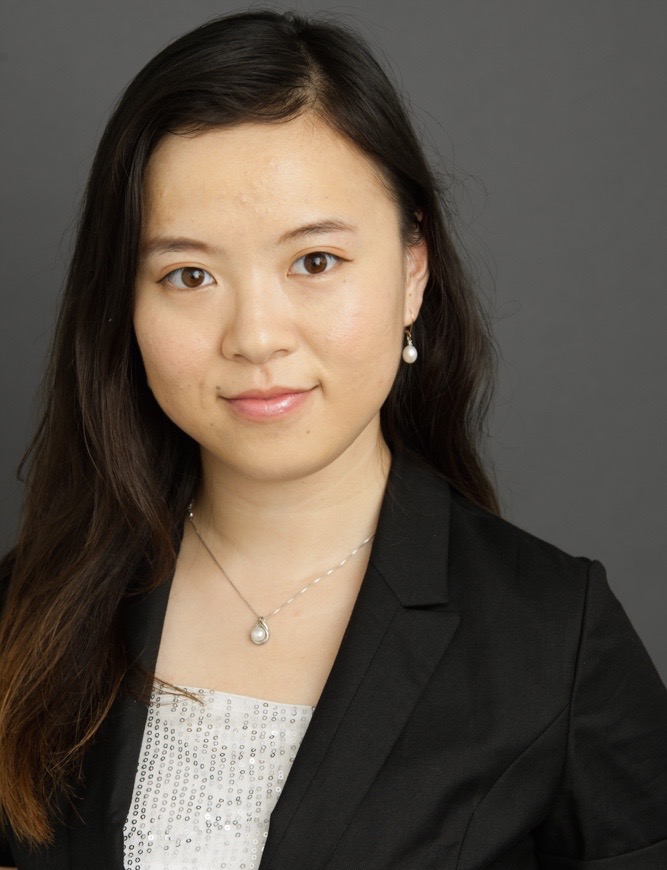
On the Interplay Between Deep Learning and Dynamical Systems
Deep Learning Dynamical Systems 北大
2023/6/16
The explosion of spatiotemporal data in the physical world requires new deep learning tools to model complex dynamical systems.
Discovering and Orienting the Edges Connected to a Target Variable in a DAG via a Sequential Local Learning Approach
Causal network Directed acyclic graph Discover causes and effects
2016/1/20
Given a target variable and observational data, we propose a sequential learning approach for discovering direct cause and effect variables of the target under the causal network frame-work. In the ap...
A Method for Inferring Label Sampling Mechanisms in Semi-Supervised Learning
Inferring Label Sampling Mechanisms Semi-Supervised Learning
2015/8/21
We consider the situation in semi-supervised learning, where the “label sampling” mechanism stochastically depends on the true response (as well as potentially on the features). We suggest a method of...
Spectral Regularization Algorithms for Learning Large Incomplete Matrices
collaborative filtering nuclear norm spectral regularization netflix prize large scale convex optimization
2015/8/21
We use convex relaxation techniques to provide a sequence of regularized low-rank solutions for large-scale matrix completion problems. Using the nuclear norm as a regularizer, we provide a simple and...
Minimizing inter-subject variability in fNIRS based Brain Computer Interfaces via multiple-kernel support vector learning
Brain Computer Interfaces Functional Near-Infrared Spectroscopy Inter-subject variability Support Vector Machines RKHS
2012/11/23
Functional Near-Infrared spectroscopy (fNIRS) is an emerging non-invasive brain computer interface (BCI) modality that measures changes in haemoglobin concentrations in the cortical tissue. To date mo...
Improving accuracy and power with transfer learning using a meta-analytic database
Meta-analysis,fMRI,multiple comparison,machine learning
2012/11/23
Typical cohorts in brain imaging studies are not large enough for systematic testing of all the information contained in the images. To build testable working hypotheses, investigators thus rely on an...
Learning Linear Bayesian Networks with Latent Variables
Linear Networks Bayesian Latent Variables
2012/11/23
This work considers the problem of learning linear Bayesian networks when some of the variables are unobserved. Identifiability and efficient recovery from low-order observable moments are established...
Learning Model-Based Sparsity via Projected Gradient Descent
Model-Based Sparsity Projected Gradient Descent
2012/11/22
Several convex formulation methods have been proposed previously for statistical estimation with structured sparsity as the prior. These methods often require a carefully tuned regularization paramete...
In this paper we discuss a novel framework for multiclass learning, defined by a suitable coding/decoding strategy, namely the simplex coding, that allows to generalize to multiple classes a relaxatio...
On polyhedral approximations of polytopes for learning Bayes nets
polyhedral approximations of polytopes Statistics Theory Bayes nets
2011/9/19
Abstract: We review three vector encodings of Bayesian network structures. The first one has recently been applied by Jaakkola 2010, the other two use special integral vectors formerly introduced, cal...
Learning Poisson Binomial Distributions
Learning Poisson Binomial Distributions Data Structures and Algorithms
2011/10/8
Abstract: We consider a basic problem in unsupervised learning: learning an unknown \emph{Poisson Binomial Distribution} over $\{0,1,...,n\}$. A Poisson Binomial Distribution (PBD) is a sum $X = X_1 +...
Learning $k$-Modal Distributions via Testing
$k$-Modal Distributions Data Structures and Algorithms
2011/10/8
Abstract: A $k$-modal probability distribution over the domain $\{1,...,n\}$ is one whose histogram has at most $k$ "peaks" and "valleys." Such distributions are natural generalizations of monotone ($...
Convergence of distributed asynchronous learning vector quantization algorithms
distributed asynchronous vector quantization algorithms
2011/1/4
Motivated by the problem of effectively executing clustering algorithms on very large data sets, we address a model for large scale distributed clustering methods. To this end, we briefly recall some ...
Optimal learning rates for Kernel Conjugate Gradient regression
Optimal learning rates Kernel Conjugate Gradient regression
2010/12/14
We prove rates of convergence in the statistical sense for kernel-based least squares regression using a conjugate gradient algorithm, where regularization against overfit-ting is obtained by early st...